Barak Turovsky (Exec In Residence): How to Evaluate Generative AI Use Cases
Why search might be a red herring and who will capture the most value in the AI stack
Join 42,000+ others who get my best writing and interviews on how to level up your product skills and build a thriving creator business.
Dear subscribers,
Today, I want to share a great framework for evaluating generative AI use cases.
Barak Turovsky is Executive in Residence at Scale Venture Partners and ex-head of product for Google Languages AI. I worked with Barak a decade ago, so naturally I had to chat with him about AI.
In the interview below, we talk about:
How to evaluate generative AI use cases
Why search might be a red herring vs. other use cases
Which companies will capture the most value in the AI stack
How to evaluate generative AI use cases
Welcome Barak! What’s your framework for evaluating generative AI use cases?
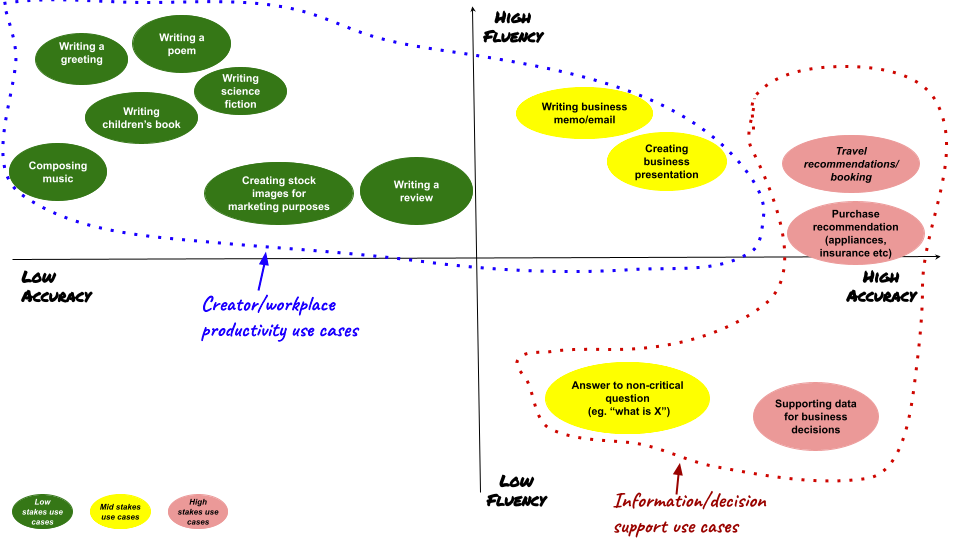
I like to evaluate use cases across two axes:
Fluency: How natural sounding the output is.
Accuracy: How correct the output is.
Here’s the breakdown: