Brian Balfour (Reforge): A Deep Dive Into How the Best AI Products Grow
The growth loops powering AI products like ChatGPT, Midjourney, Perplexity, and LinkedIn collaborative articles
Dear subscribers,
Today, I want to share a deep dive into how top AI products grow with one of the best in the business.
Brian Balfour is CEO of Reforge and host of the Unsolicited Feedback podcast. Brian has taught 1000s of people about growth so naturally, I had to ask him to deconstruct how four top AI products grew:
Why ChatGPT is investing in custom GPTs
How Midjourney grew to $200M ARR despite a high-friction Discord UX
How Perplexity can compete with Google Search
How LinkedIn collaborative articles combine AI and user growth loops
Let’s dive in.
Why ChatGPT is investing in custom GPTs
Welcome Brian! Let’s start with ChatGPT. Can you break down the growth loops that fueled ChatGPT’s rise to 100M users in just 2 months?
ChatGPT is an interesting case that I think nobody should try to follow.
With most products, you go through this sequence of:
Doing things that don’t scale to get your first few customers.
Building growth loops that are more sustainable over time.
ChatGPT is one of those rare products that went from 0 to 100M users just by building a 10x better product than what came before.
Because of that, I think the primary driver of ChatGPT’s growth is word of mouth. People shared their ChatGPT conversations on social which then got mainstream media attention. The product truly captured lightning in a bottle.
Why do you think this is such a dangerous example to follow?
It’s dangerous because founders often think that they have lightning in a bottle too with their product. But 99 out of 100 times that’s not true.
How do you think ChatGPT can continue to grow moving forward?
AI products have a novelty effect that’s great at capturing initial attention.
But the big question is: “How fast can you improve engagement and retention before the novelty effect wears off?”
I’ve seen data that AI products are retaining at a much worse rate than non-AI products.
ChatGPT might be an exception, but I think the team is likely still focused on engagement and retention.
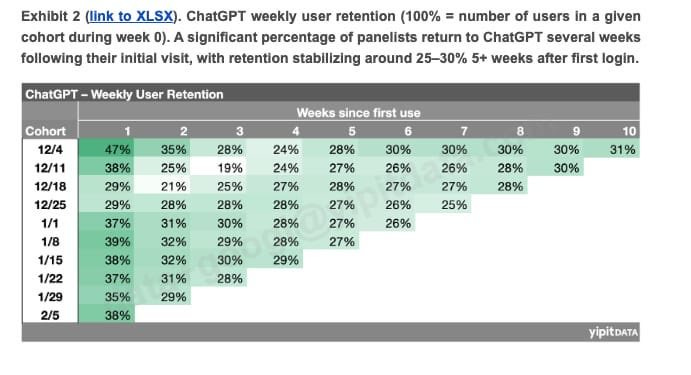
Yeah, I can only be part of a handful of group chats with real people. So I wonder how many AI chatbots people will talk to regularly.
With AI tools, I think input and output friction really matters:
Input friction is how much effort is required to get my desired output. In ChatGPT’s case, I think the input friction is quite high. You need to give it long and detailed prompts to get the best results.
Output friction is how much effort is required to take ChatGPT’s output and make it useful. The thing is, ChatGPT is such a broad tool that it can be used for many things. That’s also why I think OpenAI is investing in AI agents that can just do the task for you.
So despite its success, I think ChatGPT still has high input and output friction.
Just compare it to a product like Github Copilot that autocompletes code for you. The latter probably has better retention than ChatGPT. By the way, I think this is also why OpenAI is investing in custom GPTs.
Can you talk more about that? OpenAI first tried plugins which didn’t work. They’re now encouraging everyone to build custom GPTs. How will this improve retention?
People compare plugins to custom GPTs, but I think they serve different purposes:
Plugins were focused on increasing ChatGPT’s capabilities (e.g., PDF reader, video summaries). They fell flat because the error rate was just too high.
Custom GPTs, on the other hand, are all about lowering the input friction. New users can use custom GPTs instead of writing long, detailed prompts themselves.
For example, here’s a great prompt to learn about any topic:
You're here to help me learn about any subject I'm interested in, breaking down complex topics into understandable parts, much like Richard Feynman's teaching method.
Your goal is to explain technical details clearly, using analogies and simple language to make the subject accessible. You'll start by explaining the topic in detail, and then you'll ask me about my familiarity with any prerequisite knowledge to ensure I have a solid foundation.
If there are gaps in my understanding, you'll help fill those in with further explanations, again using analogies where helpful. We can use illustrations to aid understanding, and you'll test my grasp of the concepts with specific, technical questions.
This iterative process ensures I fully comprehend the subject at hand.
New ChatGPT users won't know how to write prompts like this. With custom GPTs, they can just use Siqi Chen’s Universal Primer GPT instead.
So to recap, I think:
Custom GPTs empower power users to reduce the input friction for new users.
It’s a play to improve retention.
I think custom GPTs can increase power user retention as well. I’ve put a lot of effort into making GPTs to help me edit blog posts, act as a private coach, and more. It’ll be hard for me to switch to another platform.
Yes, you’re right. The GPT Store incentivizes power users to turn their best prompts into creations that make it easier for casual users to get the best out of the product.
So broadly speaking, what kind of moats does ChatGPT have compared to new entrants like Google Gemini and Microsoft Copilot?
ChatGPT and similar products are horizontal tools. The benefit is that they can be used for many things, but the drawback is that it’s often hard for new users to understand what to use them for.
So I think OpenAI is building a growth loop where:
Creators build GPTs to reach ChatGPT’s 100M users.
New users use these GPTs to get the most out of the product.
It’s also worth comparing ChatGPT to horizontal tools that grew in the pre-AI world. Loom, Notion, and Figma all have huge template libraries that make it easy for new users to try different use cases.
I think GPTs will follow a similar path. But the jury is still out on whether they have enough creators making GPTs to replicate that approach.
Do you think this approach will help OpenAI build a data moat as well?
Yes, it certainly accelerates OpenAI’s data loops.
But I think when it comes to moats and defensibility:
The real race is who gets to AGI first.
I don’t think any of these AI products are thinking about network effects or other traditional defensibility mechanisms.
Everything that OpenAI is doing is probably being run through an internal strategic filter of “How does this get us to AGI faster?”
Let’s face it — the first product that gets to AGI is going to have the ultimate moat.
How Midjourney grew to $200M ARR despite a high-friction Discord UX
Let’s talk about MidJourney next. We’ve discussed how important new user activation is. Yet, MidJourney has relied on Discord as its primary interface.
It took me a while to figure out how to even generate an image. Why do you think they pursued this strategy and why has it worked out so far?
Keep reading with a 7-day free trial
Subscribe to Creator Economy by Peter Yang to keep reading this post and get 7 days of free access to the full post archives.